Article reprint from

QOMPLX, the company that created a cloud-based Enterprise Operating System based on industrial-scale analytics, machine learning and simulation modeling, is not one to shy away from complexity. They seem to thrive on it. As they advertise on their site, their OS offers “just-in-time, just-in-context, and just-in-place decision-making for the world’s most demanding problems.”
However, complex does not have to mean complicated. These two words, which are used interchangeably, actually represent quite different realities. A system can be complex, but not complicated, which implies difficulty. The Internet, for example, is a highly complex system, but it’s not complicated to use. In the context of data analytics system that drive decision making, it’s ideal to have an inverse relationship between complexity and complication.
QOMPLX is now applying its enterprise OS to insurance, one of the most complex arenas in the business world. Their goal is to avoid making a complex situation needlessly complicated. They’re offering a number of different platforms and services for insurance carriers and agencies, including a Managed General Agency (MGA) solution. In this context, QOMPLX is even offering underwriting services themselves.
“Insurance has always been about deep data analysis, going back to the 18th century,” explained Alastair Speare-Cole, President & General Manager of the Insurance Division of QOMPLX. “It’s about estimating risk and potential losses. Today, we have so much more data to use in making underwriting decisions—so much that it can easily overwhelm the process. This is the problem we’re solving.”
To illustrate his point, Speare-Cole described the challenge of underwriting multi-line insurance. “If you’re just insuring for one line, like autos, your risk analysis is pretty straightforward,” he said. “Where it can get very complicated is figuring out how to come up with a single premium to cover E&O, physical damage, defamation and on and on. Traditionally, this process has led to a degradation of the carrier’s economics or a degradation of the underwriting standards. There’s just too much data to look at from multiple sources.”
To avoid the complications of analyzing multiple, massive data sources, the QOMPLX context-driven decision platform for insurance features what Speare-Cole “human-machine teaming,” a form of AI-assisted decision making. “The machine can make the preliminary decision based on ingesting and analyzing more data than a human being could ever hope to manage—but the human still has the option of saying yes or no. The machine could say ‘this is outside our limits, so I’ve declined to cover the risk,’” Speare-Cole added.
The decision processes enabled by the QOMPLX product are relevant across the insurance industry. However, they’re particularly useful in cyber insurance. This is a new field, where there is not enough historical loss data for traditional underwriting techniques. With its ability to cut down on complications in highly complex data analytics scenarios, QOMPLX is poised to have an impact on cyber underwriting. Speare-Cole offered a perspective on mitigating cyber risk through insurance in our recent infographic.
Infographic: Cyber Insurance Drivers of Loss
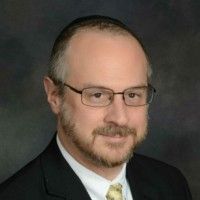
About the Author
Hugh Taylor
Hugh Taylor is a Certified Information Security Manager (CISM). In addition to editing Journal of Cyber Policy, he writes about cybersecurity, compliance and enterprise technology for such clients as Microsoft, IBM, SAP, HPE, Oracle, Google and Advanced Micro Devices. Prior to launching his freelance writing career, he served in executive roles at Microsoft, IBM and several venture-backed technology startups.